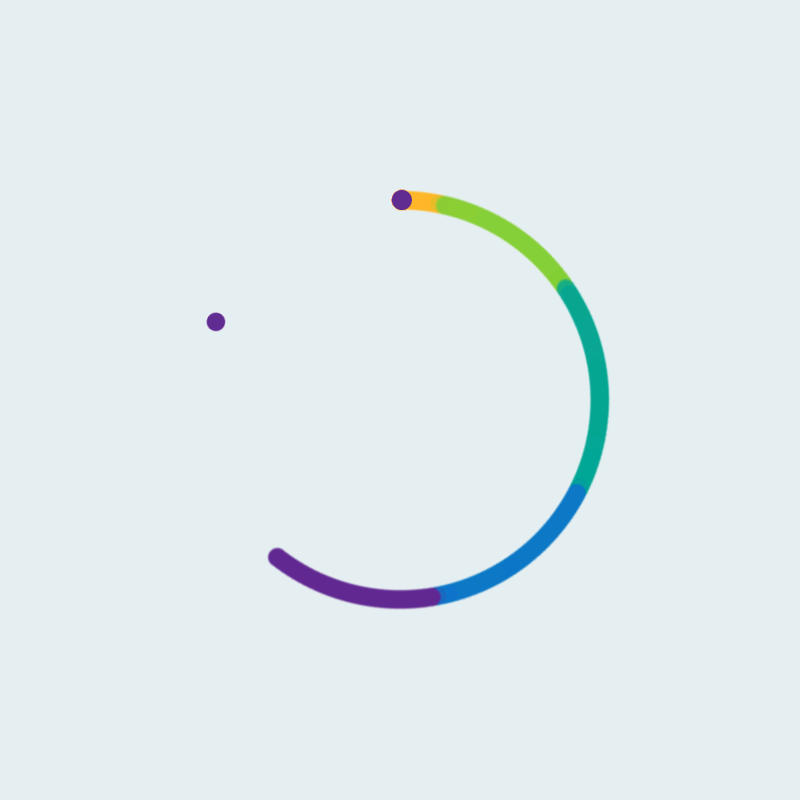
Global data analytics market was valued at USD 43.59 Billion in 2019 and it is expected to reach USD 209.26 Billion in 2025 growing at a CAGR of 29.88% over the forecast period.
With the help of data analytics, organizations can now influence data to extract valuable insights, which are used to create illegal decisions. In the past few years, data analytics has been roughly used to authorize organizations to make the most profitable business choices.?Additionally, the rapidly increasing volume and complexity of data are due to growing mobile data traffic, cloud-computing traffic and burgeoning development and adoption of technologies including IoT and AI, which is pouring the growth of big data analytics market. Over 2.5 quintillion bytes of data created every day. Data is produced by every click, swipe, share, search, and stream, proliferating the demand for big data analytics market worldwide.
However, the need for data science has manifested across all industries since almost every business is striving to establish a strong digital presence.?The flow in spending for data science solutions, talent within the industry, and successful implementations demonstrate that businesses understand the incredible impact insights have on organizational performance.?
Trends –
Data is Becoming Strategic –
Data managers are seeing their mandates extend well beyond their original roles as administrators and troubleshooters in managing and securing day-to-day transactions. It’s now about leveraging information to make strategic, operational, and tactical decisions that result in increased revenue, improve operational efficiency, and enhance customer experience.
Customer Service is Being Driven by Data –
Superior customer experience, or CX, has increasingly been acknowledged as a competitive differentiator and is driving a closer alliance between marketing, customer service, and IT or data managers. This is due to CX almost wholly depends on the intelligence and insights provided by data. In addition, personalization, in which data is sourced to deliver targeted customer experiences, has emerged as a leading priority on business leaders’ agendas. The “experience” aspect doesn’t just stop at customers either, data needs to deliver highly personalized experiences to end users such as employees.
More Self-Service –
There is a growing emphasis on enabling end users to create their own queries to ask any question at any time of their data—without having to requisition reports from their IT departments. Vendors recognize the need for such flexibility, as well as the challenge of finding data across enterprises, and are responding with user-empowerment tools and offerings. From an organizational point of view, enterprises need to ensure that the process for acquiring and integrating this data is well-managed and well-governed, with policies and frameworks that assure the information users generate is trustworthy and reliable.
The Drive toward Data Quality Intensifies –
As data has become ever-more critical to the business, the need for decision makers to be able to trust their data has grown. Data quality is a process that begins as data sources are identified and accessed, extending to managing and storing the data. There have long been robust tools and applications on the market to help ensure data quality at the granular level, in terms of reduplication and cleanliness, but an effective data quality effort also needs to encompass its timeliness and the governance policies that surround it. Decision makers need to be assured that their real-time analytics and AI systems are employing the highest-quality data available.
DataOps and DevOps –
Not only does data need to be readily available for decision making, but a process needs to be put in place that ensures that it is moved and processed on a continuous basis, as automatically as possible. That’s why many enterprises are turning to emerging practices such as the DataOps and DevOps models of continuous integration and continuous delivery. DevOps is concerned with the process for developing and delivering application releases, while DataOps is an automated, process-oriented methodology to improve the quality and reduce the cycle time of data analytics. Lately, both of these methodologies are being applied to enable organizations to move quickly to have access to the latest algorithms and data to stay on top of their markets.
AI and Machine Learning -
No discussion of the power of data analytics in 2019, of course, can take place without including AI and machine learning. For starters, AI is replacing standard BI reporting as we’ve known it for decades, with real-time insights and updates on changes or developments within selected areas of the business. With AI, of course, many analytics-driven decisions can be made and executed without human intervention. At the same time, at a higher level, AI is assisting decision makers in understanding what data is telling them.
Bots and Digital Workers –
Another trend on the horizon for data analytics is the use of robotic process automation and the rise of digital workers. As more “bots” take on the day-to-day tasks of back-office work, such as managing workflows or searching files, data analytics is increasingly laying the groundwork for their intelligent performance.
Data Ecosystems Expanding –
Data environments are no longer insular systems contained within corporate walls. The ability to deliver and act on data-driven insights is increasingly amplified by connected ecosystems of partners, customers, and other constituencies. Data-driven enterprises are learning to bring together expertise and knowledge from both inside and outside their corporate walls to deliver growth and innovation.
Augmented Data Management –
With technical skills in short supply and data growing exponentially, organizations need to automate data management tasks. Vendors are adding machine learning and artificial intelligence (AI) capabilities to make data management processes self-configuring and self-tuning so that highly skilled technical staff can focus on higher-value tasks.
This trend is impacting all enterprise data management categories, including data quality, metadata management, master data management, data integration and databases.
Graph Analytics –
Business users are asking increasingly complex questions across structured and unstructured data, often blending data from multiple applications, and increasingly, external data. Analyzing this level of data complexity at scale is not practical, or in some cases possible, using traditional query tools or query languages such as SQL.
Graph analytics is a set of analytic techniques that shows how entities such as people, places and things are related to each other. Applications of the technology range from fraud detection, traffic route optimization and social network analysis to genome research. Gartner predicts that the application of graph processing and graph databases will grow at 100% annually over the next few years to accelerate data preparation and enable more complex and adaptive data science.
Commercial AI and Machine Learning -
Open-source platforms currently dominate artificial intelligence (AI) and machine learning and have been the primary source of innovation in algorithms and development environments. Commercial vendors were slow to respond, but now provide connectors into the open-source ecosystem. They also offer enterprise features necessary to scale AI and ML, such as project and model management, reuse, transparency and integration, capabilities that open-source platforms currently lack.
Increased use of commercial AI and ML will help to accelerate the deployment of models in production, which will drive business value from these investments.
Read More: